In my last post I articulated a concern about how the name adopted by this field may drive the research in one direction or another. I’ve adopted the Physiological Computing (PC) label because it covers the widest range of possible systems. Whilst the PC label is broad, generic and probably vague, it does cover a lot of different possibilities without getting into the tortured semantics of categories, sub-categories and sub- sub-categories.
I’ve defined PC as a computer system that uses real-time bio-electrical activity as input data. At one level, moving a mouse (or a Wii) with your hand represents a form of physiological computing as do physical interfaces based on gestures – as both are ultimately based on muscle potentials. But that seems a little pedantic. In my view, the PC concept begins with Muscle Interfaces (e.g. eye movements) where the electrical activity of muscles is translated into gestures or movements in 2D space. Brain-Computer Interfaces (BCI) represent a second category where the electrical activity of the cortex is converted into input control. Biofeedback represents the ‘parent’ of this category of technology and was ultimately developed as a control device, to train the user how to manipulate the autonomic nervous system. By contrast, systems involving biocybernetic adaptation passively monitor spontaneous activity from the central nervous system and translate these signals into real-time software adaptation – most forms of affective computing fall into this category. Finally, we have the ‘black box’ category of ambulatory recording where physiological data are continuously recorded and reviewed at some later point in time by the user or medical personnel.
I’ve tried to capture these different categories in the diagram below. The differences between each grouping lie on a continuum from overt observable physical activity to covert changes in psychophysiology. Some are intended to function as explicit forms of intentional communication with continuous feedback, others are implicit with little intentionality on the part of the user. Also, there is huge overlap between the five different categories of PC: most involve a component of biofeedback and all will eventually rely on ambulatory monitoring in order to function. What I’ve tried to do is sketch out the territory in the most inclusive way possible. This inclusive scheme also makes hybrid systems easier to imagine, e.g. BCI + biocybernetic adaptation, muscle interface + BCI – basically we have systems (2) and (3) designed as input control, either of which may be combined with (5) because it operates in a different way and at a different level of the HCI.
As usual, all comments welcome.
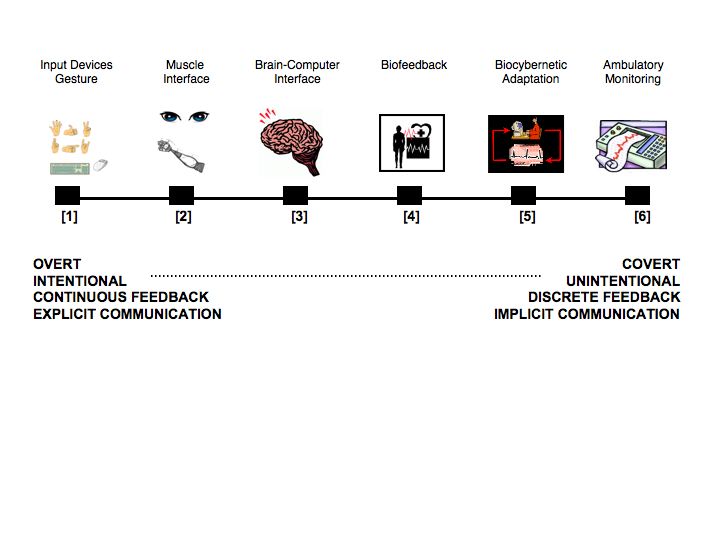
Five Categories of Physiological Computing